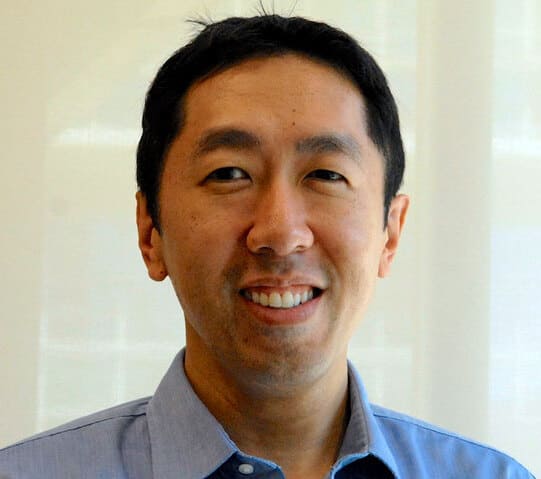
This article examines Deep Learning’s limitations and the opinions from Experts. It also explores possible solutions. These limitations include time and cost associated with labeling data and collecting it. Deep Learning should not, however, be criticized. This discussion should instead be viewed as an examination of the limitations of Deep Learning.
Experts' perceptions about deep learning limitations
One of the limitations of deep learning is that it requires a huge amount of data to train. Deep learning algorithms do poorly when there is a small amount of data. Standard machine-learning methods can improve performance even if there is a small amount of data. Deep learning techniques should be combined with unsupervised learning, which does not heavily rely upon labeled training information, to overcome these limitations.
Deep learning algorithms are multi-layered and use multiple layers to train computer programmers. Each layer applies a nonlinear transform to the input, and then uses the learned information in order to create a statistical model. This is repeated until the output has acceptable accuracy. The number and complexity of the processing layers in an algorithm determines what the term "deep".
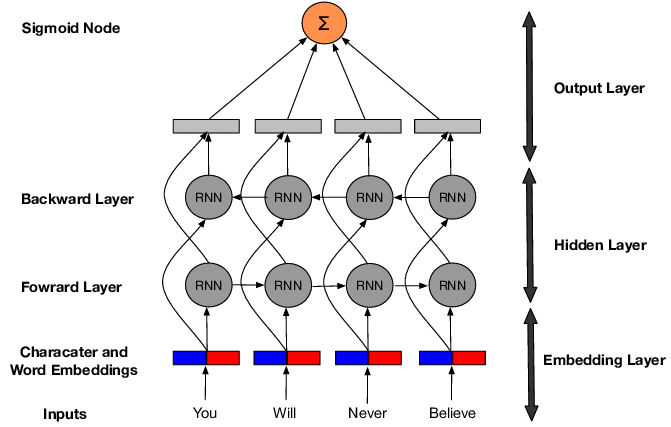
Deep learning requires enormous amounts of processing power in order to process the data. Deep learning programming, however, can create complex statistical models from unlabeled data if there are a lot of them. These devices produce huge amounts of data that are not labeled, and the internet of things is making it more popular.
Possible solutions to overcome these limitations
Deep learning offers many potential benefits but it also has its limitations. For example, it is limited in its ability to perform classification tasks without enough training data. It cannot also solve problems that require reinforcement learning or rule-based programing. These limitations can be overcome by researchers who are now focusing their attention on AI's neuroscience.
Deep learning relies very little on human input. Because of this, it requires a lot of data and lots of computing power. Training time can be reduced significantly with high-performance GPUs and the right infrastructure. Deep learning models are much faster than human operators. Furthermore, their quality never decreases as the training dataset grows.
Deep learning is still very young, but it has already shown great promise in many areas. One of the most promising areas is gene expression prediction. A deep neural network with three hidden layers outperformed linear regression. These methods could also prove to be clinically applicable, as they can utilize fluorescence microscopy data for identifying cellular states.
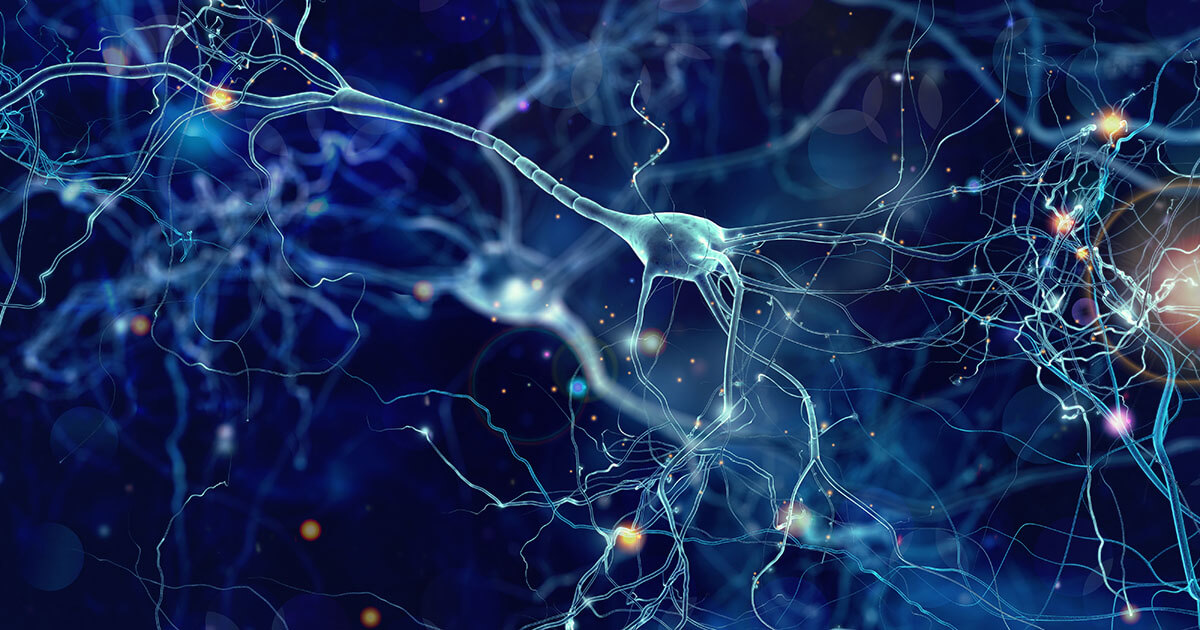
Data collection and labeling can cost and take time
Collecting and labeling data for deep learning models can be costly and time-consuming. You might consider hiring experts to label the open-source datasets. Experts such as these are highly paid, and they dedicate a lot time to the task. However, their fees are high and could extend the deadline. To scale up the workforce, it is expensive to hire additional labelers.
Another cost-effective option for labeling data is crowdsourcing. You can reward each assignment with a reward. A $100 reward can be given for labeling more than 2000 images. You can take on up to nine tasks for this price. Crowdsourcing isn’t ideal because the data may not be of high quality.
Additional to the cost of labeling data, preparing and storing them can also be a significant expense. Annotating videos requires a lot labor. A 10-minute video that contains 18,000-36,000 frame requires 800 hours of human labor. The frame rate is 30-60 frames/second.
FAQ
Is Alexa an Ai?
Yes. But not quite yet.
Amazon developed Alexa, which is a cloud-based voice and messaging service. It allows users interact with devices by speaking.
The Echo smart speaker, which first featured Alexa technology, was released. Other companies have since used similar technologies to create their own versions.
Some examples include Google Home (Apple's Siri), and Microsoft's Cortana.
What countries are the leaders in AI today?
China has the largest global Artificial Intelligence Market with more that $2 billion in revenue. China's AI market is led by Baidu. Tencent Holdings Ltd. Tencent Holdings Ltd. Huawei Technologies Co. Ltd. Xiaomi Technology Inc.
China's government is heavily investing in the development of AI. China has established several research centers to improve AI capabilities. The National Laboratory of Pattern Recognition is one of these centers. Another center is the State Key Lab of Virtual Reality Technology and Systems and the State Key Laboratory of Software Development Environment.
China is also home to some of the world's biggest companies like Baidu, Alibaba, Tencent, and Xiaomi. All these companies are actively working on developing their own AI solutions.
India is another country that is making significant progress in the development of AI and related technologies. India's government is currently focusing their efforts on creating an AI ecosystem.
Who created AI?
Alan Turing
Turing was created in 1912. His father was clergyman and his mom was a nurse. After being rejected by Cambridge University, he was a brilliant student of mathematics. However, he became depressed. He discovered chess and won several tournaments. After World War II, he worked in Britain's top-secret code-breaking center Bletchley Park where he cracked German codes.
1954 was his death.
John McCarthy
McCarthy was born in 1928. He studied maths at Princeton University before joining MIT. There, he created the LISP programming languages. In 1957, he had established the foundations of modern AI.
He passed away in 2011.
AI is good or bad?
AI can be viewed both positively and negatively. The positive side is that AI makes it possible to complete tasks faster than ever. There is no need to spend hours creating programs to do things like spreadsheets and word processing. Instead, we can ask our computers to perform these functions.
People fear that AI may replace humans. Many believe that robots may eventually surpass their creators' intelligence. This means that they may start taking over jobs.
Statistics
- By using BrainBox AI, commercial buildings can reduce total energy costs by 25% and improves occupant comfort by 60%. (analyticsinsight.net)
- A 2021 Pew Research survey revealed that 37 percent of respondents who are more concerned than excited about AI had concerns including job loss, privacy, and AI's potential to “surpass human skills.” (builtin.com)
- That's as many of us that have been in that AI space would say, it's about 70 or 80 percent of the work. (finra.org)
- While all of it is still what seems like a far way off, the future of this technology presents a Catch-22, able to solve the world's problems and likely to power all the A.I. systems on earth, but also incredibly dangerous in the wrong hands. (forbes.com)
- According to the company's website, more than 800 financial firms use AlphaSense, including some Fortune 500 corporations. (builtin.com)
External Links
How To
How do I start using AI?
Artificial intelligence can be used to create algorithms that learn from their mistakes. You can then use this learning to improve on future decisions.
For example, if you're writing a text message, you could add a feature where the system suggests words to complete a sentence. It could learn from previous messages and suggest phrases similar to yours for you.
The system would need to be trained first to ensure it understands what you mean when it asks you to write.
Chatbots can also be created for answering your questions. So, for example, you might want to know "What time is my flight?" The bot will tell you that the next flight leaves at 8 a.m.
You can read our guide to machine learning to learn how to get going.