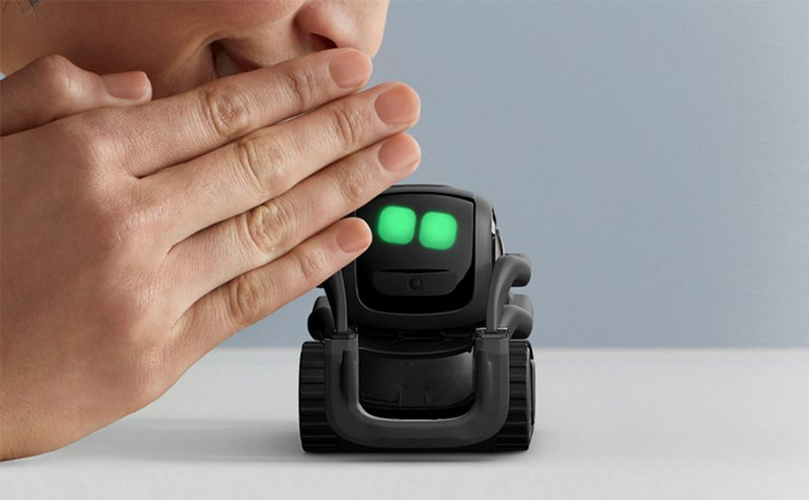
Data scientists develop the algorithms that allow machine learning to happen. Data scientists train algorithms using data, and machine learning can be applied to many other fields than data science. Machine learning includes deep learning. Data scientists are involved in the development of algorithms that make deeplearning possible. They are also capable of creating models that are not easily accessible by humans. In this article we will explore the differences between data science (machine learning) and how each can benefit your business.
Data scientists create the algorithms that make machine learning happen
Although ML and data science may not be the same thing, they are complementary and interconnected. Machine learning engineers and data scientists create the algorithms that machine learning works. A product or service's commercial value can be enhanced by teaming up with data scientists and machine learning engineers. Although they share the same projects, machine learning engineers and data scientists have different responsibilities. In the early stages of a product development process, data scientists are responsible for creating candidate machine learning models and handing them over to machine learning engineers to build the ground labels.
Machine learning algorithms make predictions using as much data as possible. The algorithm learns from humans and can recognize different features. As the algorithm learns more data, it becomes more accurate. It is necessary to manually classify the data in order to train the algorithm. This is vital to the success of the product/service. Before machine learning algorithms can work, they have to be trained with human data.
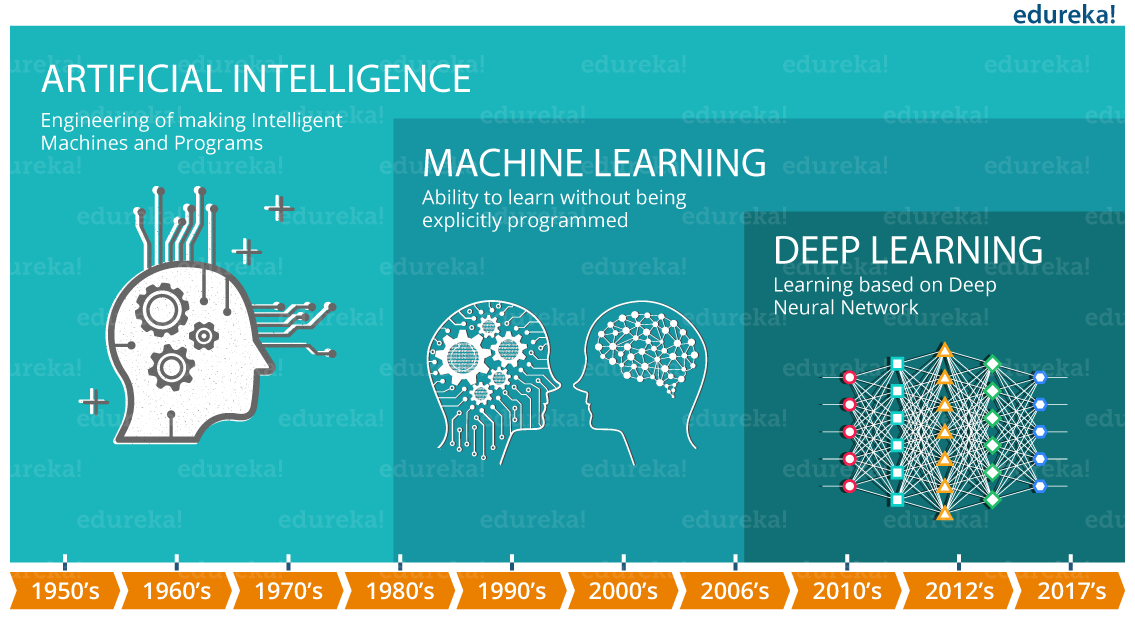
Machine learning is one subset in artificial intelligence.
Machine learning is closely related to computational statistical. Both are concerned with the analysis of data and probabilities. Machine learning uses algorithms to set up computers to carry out tasks without explicit programming. Typically, these computers are fed with structured data and 'learn' to evaluate that data over time. Some implementations mimic the functioning of the biological brain. Machine learning is also called predictive analytics.
Artificial intelligence is a vast field. However, it's a very niche area. In 2017, the DOMO company created Mr. Roboto, a robot that uses powerful analytics tools to analyze data and give insight into business development. It can detect patterns and anomalies and is programmed to play and learn games without human input. AI development is being pursued by large corporations. Machines will one day be able to think and solve logical problems with no human input.
Deep learning is a form of machine learning
Deep learning is machine learning that recognizes objects from analog inputs. Yann Lun, who was the father and founder of Convolutional Network (CNN), defined deep-learning as the creation large CNNs. These networks are able to scale with data and become more efficient over time, making them a great choice for many data-science applications. Although the technology was initially used primarily for research and scientific purposes, it has been adopted by industry since 2010.
The process of deep learning involves training an algorithm to recognize images and recognize objects based on a number of different inputs. Neural networks are composed of many layers. Each layer contains a specific input. The more layers, the more precise the classification. Deep learning employs neural networks for a wide range tasks including image recognition, medical diagnostics and autonomous vehicles.
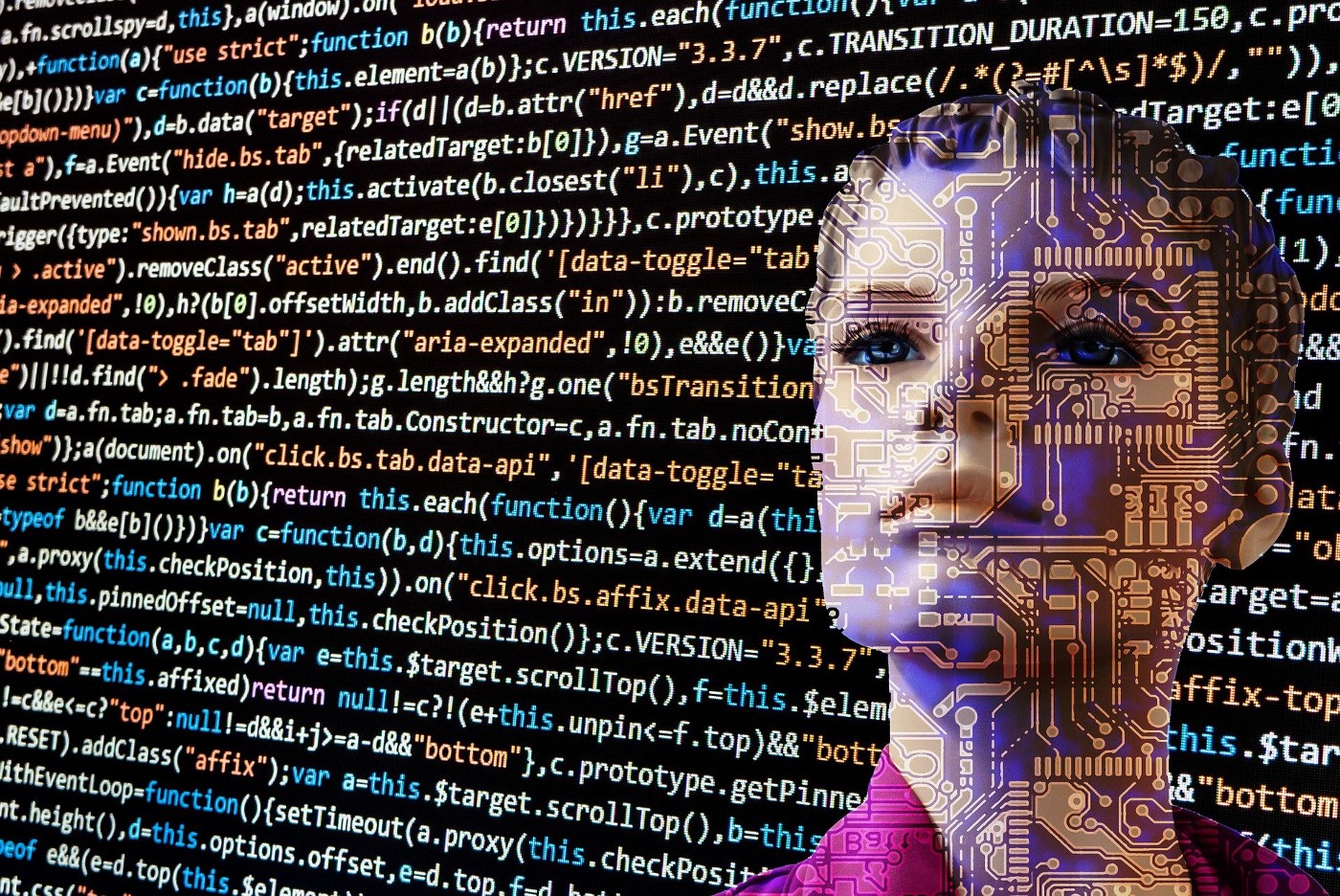
Machine learning is used in many areas beyond data science
While most people think of machine learning applications in data science as being restricted to the world of artificial intelligence, it has many other uses. Machine learning algorithms are able to flag suspicious transactions for human intervention in banking, for instance. In addition to banking, machine learning algorithms are used by smartphone voice assistants to understand human speech and make smart responses. Machine learning algorithms can be used in many industries, including entertainment and eCommerce.
It is used for speech and image recognition. The output often comes in the form of words or syllables. Some of the most popular speech recognition software are Siri, Google Assistant, YouTube Closed Captioning and many other. These technologies enable individuals to make better decisions based on the data that they collect.
FAQ
Which are some examples for AI applications?
AI can be used in many areas including finance, healthcare and manufacturing. These are just a handful of examples.
-
Finance - AI is already helping banks to detect fraud. AI can spot suspicious activity in transactions that exceed millions.
-
Healthcare – AI is used for diagnosing diseases, spotting cancerous cells, as well as recommending treatments.
-
Manufacturing – Artificial Intelligence is used in factories for efficiency improvements and cost reductions.
-
Transportation - Self-driving vehicles have been successfully tested in California. They are being tested across the globe.
-
Utilities are using AI to monitor power consumption patterns.
-
Education - AI has been used for educational purposes. Students can interact with robots by using their smartphones.
-
Government - AI is being used within governments to help track terrorists, criminals, and missing people.
-
Law Enforcement-Ai is being used to assist police investigations. Search databases that contain thousands of hours worth of CCTV footage can be searched by detectives.
-
Defense - AI can be used offensively or defensively. Offensively, AI systems can be used to hack into enemy computers. In defense, AI systems can be used to defend military bases from cyberattacks.
What is AI and why is it important?
It is predicted that we will have trillions connected to the internet within 30 year. These devices will include everything from fridges and cars. The Internet of Things is made up of billions of connected devices and the internet. IoT devices are expected to communicate with each others and share data. They will also be capable of making their own decisions. For example, a fridge might decide whether to order more milk based on past consumption patterns.
It is expected that there will be 50 Billion IoT devices by 2025. This is an enormous opportunity for businesses. But, there are many privacy and security concerns.
How will governments regulate AI?
While governments are already responsible for AI regulation, they must do so better. They must make it clear that citizens can control the way their data is used. And they need to ensure that companies don't abuse this power by using AI for unethical purposes.
They should also make sure we aren't creating an unfair playing ground between different types businesses. A small business owner might want to use AI in order to manage their business. However, they should not have to restrict other large businesses.
What is the future of AI?
Artificial intelligence (AI), the future of artificial Intelligence (AI), is not about building smarter machines than we are, but rather creating systems that learn from our experiences and improve over time.
This means that machines need to learn how to learn.
This would enable us to create algorithms that teach each other through example.
Also, we should consider designing our own learning algorithms.
It's important that they can be flexible enough for any situation.
Statistics
- That's as many of us that have been in that AI space would say, it's about 70 or 80 percent of the work. (finra.org)
- By using BrainBox AI, commercial buildings can reduce total energy costs by 25% and improves occupant comfort by 60%. (analyticsinsight.net)
- In the first half of 2017, the company discovered and banned 300,000 terrorist-linked accounts, 95 percent of which were found by non-human, artificially intelligent machines. (builtin.com)
- According to the company's website, more than 800 financial firms use AlphaSense, including some Fortune 500 corporations. (builtin.com)
- In 2019, AI adoption among large companies increased by 47% compared to 2018, according to the latest Artificial IntelligenceIndex report. (marsner.com)
External Links
How To
How to Set Up Siri To Talk When Charging
Siri can do many things, but one thing she cannot do is speak back to you. This is because your iPhone does not include a microphone. If you want Siri to respond back to you, you must use another method such as Bluetooth.
Here's how you can make Siri talk when charging.
-
Under "When Using assistive touch" select "Speak When Locked".
-
Press the home button twice to activate Siri.
-
Siri will speak to you
-
Say, "Hey Siri."
-
Just say "OK."
-
Tell me, "Tell Me Something Interesting!"
-
Say "I'm bored," "Play some music," "Call my friend," "Remind me about, ""Take a picture," "Set a timer," "Check out," and so on.
-
Speak "Done"
-
Say "Thanks" if you want to thank her.
-
If you're using an iPhone X/XS/XS, then remove the battery case.
-
Reinstall the battery.
-
Place the iPhone back together.
-
Connect the iPhone to iTunes.
-
Sync the iPhone
-
Enable "Use Toggle the switch to On.